A novel artificial intelligence (AI) tool, validated using NHS eye imaging datasets through INSIGHT, could transform the efficiency of screening for Diabetic Retinopathy (DR) — a potentially sight-threatening condition affecting a third of the 4.4 million people estimated to have diabetes in the UK.
Researchers at King’s College London have used anonymised NHS eye data from over 100,000 people with diabetes to build an AI model that can accurately predict who is at a high risk of developing sight-threatening Diabetic Retinopathy (DR) up to three years in advance.
DR is an eye condition that affects around one in three people with diabetes, and a leading cause of vision loss in working-age adults.
Anyone aged 12 years or older diagnosed with diabetes under the NHS is asked to attend an annual eye check for DR with the NHS Diabetic Eye Screening Program (DESP), which screens around 3.2 million people at a cost of over £85 million pounds per year in England alone.
The AI model could serve as a tool to enable individualised DESP screening by predicting if an individual is at low or high risk of developing sight-threatening DR in one year, two years or three years using images from the back of the eye – a capability that the NHS does not currently have.
If implemented, individualised screening could reduce the screening burden for people at low risk of vision loss, while ensuring individuals at a high-risk of vision loss are seen urgently. This approach could save the NHS millions of pounds, and thousands of appointments every year.
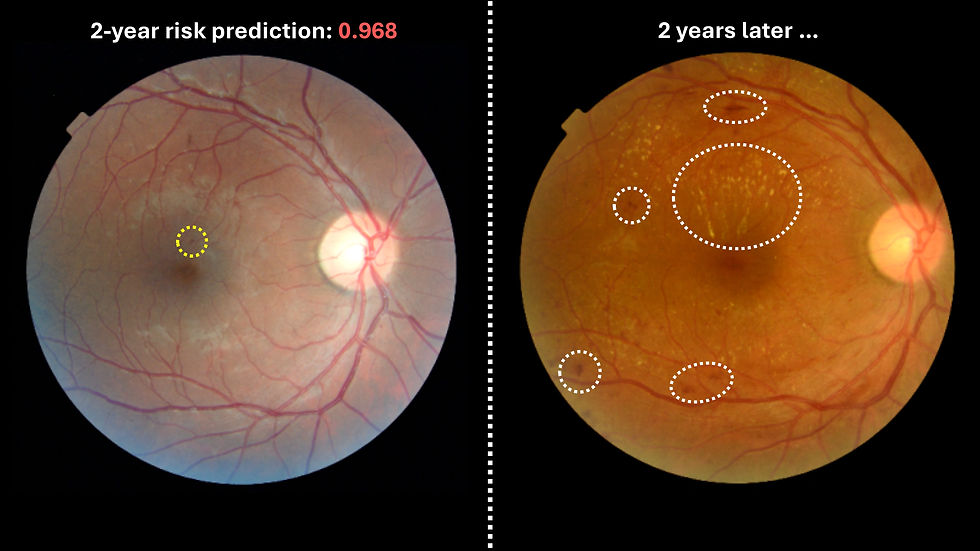
Addressing health inequity in AI
In developing the AI model, the researchers used over 1.2 million retinal images from people with diabetes from South East London DESP. To ensure that the model was robust enough to work accurately on a diverse range of individuals, it was validated on a dataset of around 70,000 images from the INSIGHT Health Data Research Hub.
INSIGHT is an NHS-led initiative and the world’s largest ophthalmic bio-resource of retinal images linked to clinical data. The images are routinely collected from patients in two of the UK’s most diversely populated cities served by Moorfields Eye Hospital and University Hospitals Birmingham NHS Foundation Trust. The INSIGHT dataset was curated from over 200,000 patients from the Birmingham, Solihull and Black Country DESP, the largest urban screening programme in Europe.
As a next step toward potential adoption, the research team plans to conduct a prospective clinical trial to assess if the AI model is safe, efficacious and cost-effective for use within DESP. The trial aims to include data from over 50,000 people with diabetes. If successful, the trial could provide evidence to support the implementation of predictive AI in the NHS DESP.
The research has been published in Nature Communications Medicine.
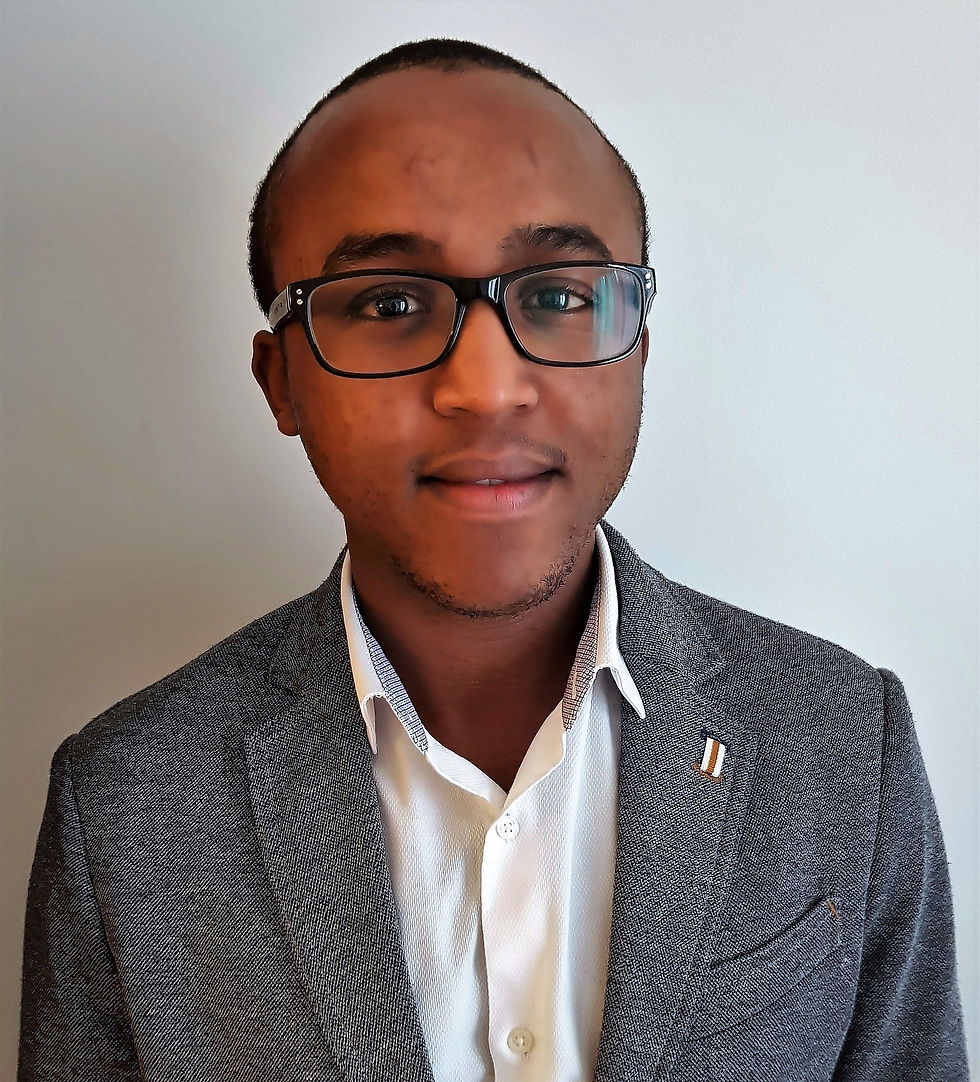
Study lead Dr Paul Nderitu said:
“The data for developing our AI model reflected the catchment of the South East London screening service, where around 30% of attendees are of Afro-Caribbean ethnicity. However, only around 6% of attendees in that area are from the South Asian community. We know that people of South Asian ethnicity have a higher risk of developing diabetes-related eye disease. Therefore, validating our AI model with the diverse INSIGHT dataset, in which 25% of people are of South Asian ethnicity, was critical to ensuring that our model works across a diverse group of people with diabetes.”
Pearse Keane, Professor of Artificial Medical Intelligence at UCL’s Institute of Ophthalmology and Director of INSIGHT, said:
“This is an exciting use case for the value of curated NHS eye data in research for the benefit of patients and the wider healthcare system. Although there are AI models that can detect the presence of diabetic eye disease with the accuracy of a retinal specialist, this new model breaks ground in predicting the risk of developing sight-threatening diabetic eye disease up to three years in the future.”
Professor Timothy Jackson PhD, FRCOphth, Consultant Ophthalmic Surgeon at King's College Hospital and Professor of Retinal Research at King’s College London, said:
"This project shows the enormous value of collating, curating and sharing routinely collected clinical data. It also shows the way ahead, in terms of how AI might move from hype to tangible patient benefit, subject to further clinical studies.”
Professor Christos Bergeles, Professor of Surgical Robotics at the School of Biomedical Engineering & Imaging Sciences, King’s College London, said:
“It has been a pleasure to work on this clinically driven project that leveraged the cross-cutting capabilities of the Faculty of Life Sciences & Medicine at King’s. Using AI to predict the incidence of retinal disease due to diabetes has tremendous social and economic prospects. AI can modernise diabetic screening programmes without sacrificing their current ability to prevent sight loss.”
The AI model development and validation was supported by funding grants from Diabetes UK, King’s College Hospital Charity and the Wellcome/EPSRC Centre for Medical Engineering.
Comments